Table Of Content
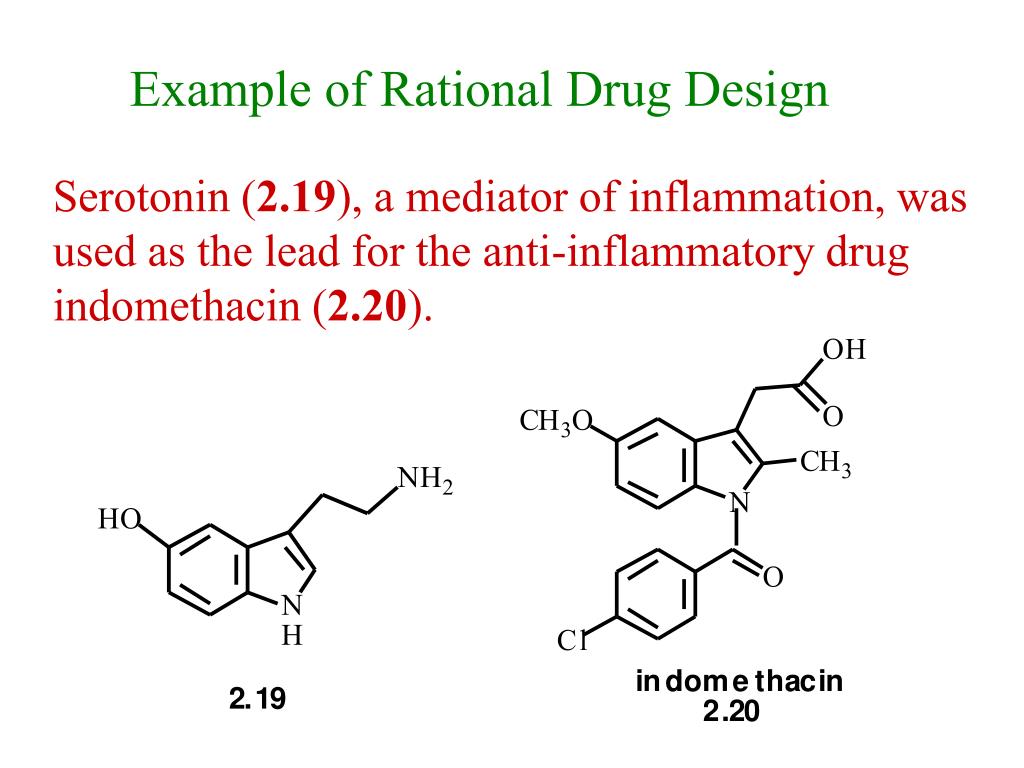
Dr. Gilson is currently on the faculty of UC San Diego’s Skaggs School of Pharmacy and Pharmaceutical Sciences. Current research in the field covers a variety of topics from the development of therapeutics for neurodegenerative diseases, to understanding the 'interactions between the cannabinoid receptor and its cytosolic regulatory proteins'. Faculty members with an interest in the field of drug development and design include the head of the department, Amy Anderson.
The Molecular Biological Mechanism of Hydrogen Therapy and Its Application in Spinal Cord Injury
However, the utilization of transfer learning and reinforcement learning in CLMs entails additional machine learning steps, which can pose challenges in terms of speed and seamless integration within the design-make-test-analysis cycle in medicinal chemistry14,15,16. Furthermore, transfer learning can be particularly challenging when applied to a single fine-tuning molecule7,17. It may also present difficulties in structure-based design applications that rely on explicit information about the protein binding site18,19,20,21.
Enter Xaira, with $1bn for its AI in drug discovery platform - pharmaphorum
Enter Xaira, with $1bn for its AI in drug discovery platform.
Posted: Wed, 24 Apr 2024 10:54:10 GMT [source]
Professional development
The graph-to-graph models would extend the capability to incorporate 3D information for structure-based molecular design, while sequence-to-sequence models would be limited to protein sequence information. Furthermore, the drug-target interactome could be expanded to include additional targets beyond those considered in the presented study. For example, RNA binding sites, protein surface binders such as molecular glues or certain macrocycles, or protein-protein interactions could be included. This expansion of the interactome would enable the exploration of distinct design possibilities and target-specific applications.
Regulatory and Quality Sciences Graduate Programs
There are a total of 12 Certificate programs available in Quality Assurance and Regulatory Affairs, including Global Pharmacovigilance, Medical Devices, and Biopharmaceuticals and Generic Drugs. The six full-time faculty members of the program have a combined experience of 100 years in the pharmaceutical and biotech fields. Additionally, Drexel University Online maintains partnerships with more than 500 businesses, including numerous health systems, for internships, networking, and potential careers for students. Current director of the Forensic Sciences and Pharmaceutical Chemistry Programs is Dr. Tebbett.
This is part of our ongoing commitment to ensure FHE Health is trusted as a leader in mental health and addiction care. The effects of using K2 can include acute kidney damage, anxiety, convulsions, hallucinations, giddiness, increased heart rate and blood pressure, organ damage, paranoia, panic attacks, and death due to heart attack. As more individuals became addicted to opioids, they often had to turn to street drugs to find a supply. U seems very appealing because of its potency and the strong high it produces, but that also comes with increased risks. Modeling In the literature it can be often found that chemists have a preference for partial least squares(PLS) methods, since it applies the feature extraction and induction in one step. The error includes model error(bias) and observational variability, that is, the variability in observations even on a correct model.
Deep learning driven de novo drug design based on gastric proton pump structures Communications Biology - Nature.com
Deep learning driven de novo drug design based on gastric proton pump structures Communications Biology.
Posted: Tue, 19 Sep 2023 07:00:00 GMT [source]
3. Speeding up Screening and Design: Artificial Intelligence in Drug Discovery
Cellular permeability was confirmed in the P-glycoprotein (Pgp) efflux assay for compounds 1 and 2, revealing values of 1.6 (15 nm ⋅ sec−1) and 1.2 (60 nm ⋅ sec−1), respectively. The observed efflux ratio indicates that compounds 1 and 2 are only interacting weakly with the Pgp transporter and thus, hold high potential to reach multiple cell and tissue types following a systemic application. Moreover, the unbound fractions of compounds 1 and 2 were determined at 0.42% and 0.21%. Such low unbound fractions are attributed to the negatively charged carboxylic acid, similar to other drug-like molecules containing carboxylic acid groups41.
This performance difference could be attributed to the complexity of the input and the availability of training data. Whereas a small-molecule graph typically comprises up to 200 edges describing covalent bonds, protein binding sites represented by 3D graphs are considerably larger with an average scaling factor of 60. Furthermore, the ligand-based data set used in the study consisted of around 501 k distinct bioaffinity values, whereas only about 236 k bioaffinities were accessible to the structure-based training procedure. This disparity in training data availability may contribute to the superior performance of the ligand-based design models. Nevertheless, it is important to acknowledge that one benefit of structure-based design applications is their flexibility in not mandating exceptionally high-quality query ligands for the generation of molecules.
The major achievement in the modern drug design is the development of in silico modelling technologies, applied for virtual screening, compound design, energy calculations, SAR and QSAR analysis, ADME modelling, modelling of drug–target interactions. In order to be applied all these advanced technologies, the molecular structure should be encoded numerically [31]. The encoded structures can be analysed, searched, visualized and compared with other structures. The structures could be encoded by binary strings, smiles strings, 2D graphs and 3D structures. The 3D molecular modelling and visualisation also are considered among the greatest achievements in drug design technologies.
Therefore in practice it still takes several iterations of design, synthesis, and testing before an optimal molecule is discovered. On the other hand, computational methods have accelerated discovery by reducing the number of iterations required and in addition have often provided more novel small molecule structures. The created data space is then usually reduced by a following feature extraction(see also dimensionality reduction). The following learning method can be any of the already mentioned machine learningmethods, e.g. support vector machines. An alternative approach uses multiple-instance learning by encoding molecules as sets of data instances, each of which represents a possible molecular conformation. A label or response is assigned to each set corresponding to the activity of the molecule, which is assumed to be determined by at least one instance in the set (i.e. some conformation of the molecule).
This approach to drug discovery is sometimes referred to as structure-based drug design. The first unequivocal example of the application of structure-based drug design leading to an approved drug is the carbonic anhydrase inhibitor dorzolamide, which was approved in 1995. The combination of DRAGONFLY with scoring functions incorporating compound synthesizability, novelty, and bioactivity towards one or multiple targets using various descriptors was demonstrated to be feasible.
ARF1 depletion apparently caused lipid droplet accumulation, consistent with the reported data56,57, and the addition of rabeprazole had little effect on lipid droplet formation on this basis (Fig. 7g). In addition, rabeprazole failed to suppress tumor growth or affect the immune response in ARF1-knockdown CT26 transplanted tumor model (Fig. 7h, i). Taken together, these data suggested that rabeprazole induced an antitumor immune response through lipid metabolism, which is dependent on ARF1.
Lately, in the analyses have been involved methods such as principal component analysis [35] and partial least squares [36]. Chemometrics evolved into chemoinformatics including molecular modelling, chemical information and tools and algorithms to handle the enormous amount of data coming from the combinatorial chemistry and HTS [37]. At the same time, bioinformatics also emerged to organise and analyse biological data, the amount of which increased dramatically, especially after deciphering the human genome. Nowadays, the structure–activity relationships are analysed by machine learning methods such as random forest, decision trees, neural networks, k nearest neighbours, etc. [38,39]. Many authors classify these methods as “black box” methods because they are not able to distinguish immediately between relevant and irrelevant for the activity descriptors as the regression methods do [39]. However, they are very good in predictions and classifications, significantly better than the statistical analyses and regression models.
We also performed a cellular thermal shift assay (CETSA), and the results supported that iRNF directly binds to and thermally stabilizes the RNF130 proteins (Supplementary Fig. 4d). 4e, KD of iRNF binding with RNF130 was 1.23 μM, ΔG and ΔH were −33.80 kJ/mol and −7.31 kJ/mol respectively, the stoichiometry of binding (N) is 1.01. Compared with other tools, iRNF was highly ranked and discovered only by TransformerCPI2.0 (Supplementary Table 11).
Both compounds have shown a clear profile not reaching ≥50% inhibition or binding at a concentration of 10 μM with the exception for PPARγ (Tables S9–S12). HEK293T cells were seeded at the indicated number per well in DMEM-high glucose, complemented with glutamax, pen-strep, and 10% FBS, in a total of 40 μl of medium. Compounds were added to the cells at the indicated concentrations, resulting in a final Dimethylsulfoxid (DMSO) concentration of 0.2%. At the specified time point, the medium was carefully removed from the vessel, leaving only 2 μl in the wells.
No comments:
Post a Comment